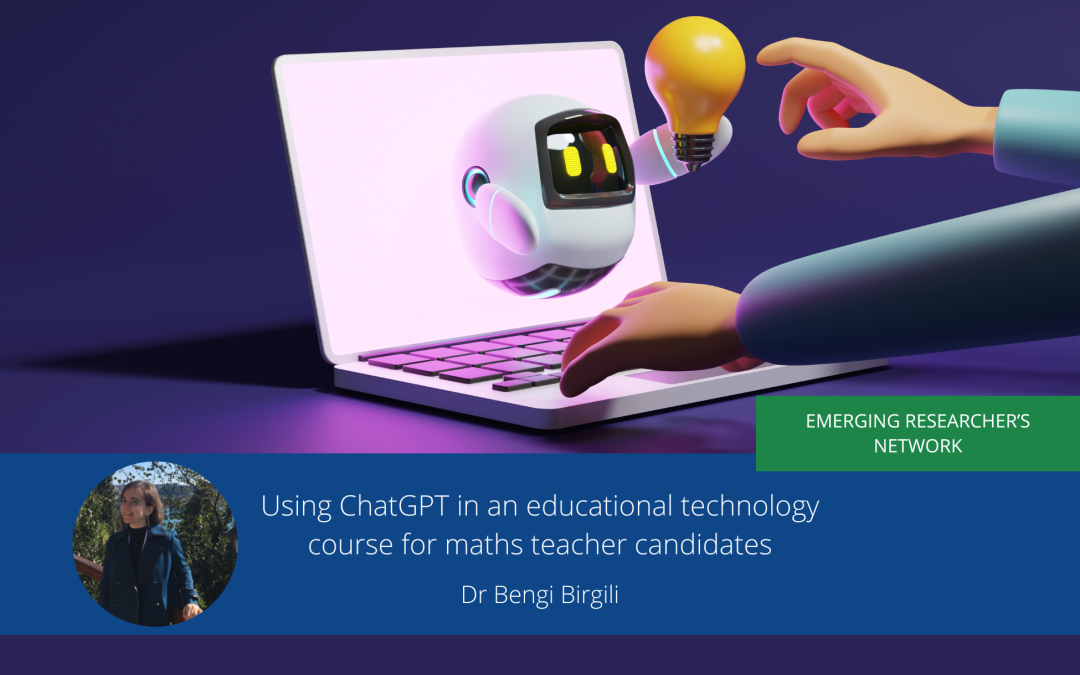
Using ChatGPT in an educational technology course for maths teacher candidates
There has been a lot of discussion in educational research circles about the use of AI in education, in particular, ChatGPT. We asked doctoral research assistant, Bengi Birgili to tell us about how she is using (and teaching the use of) ChatGPT in the classroom. Dr Birgili introduced a fully flipped university context from the view of a researcher instructor. In this post, she explains how she and her students used ChatGPT in an instructional technology course offered in the Spring 2023 semester. This blog post includes not only her ideas and experiences but also those of 30 pre-service teachers studying in the mathematics education department in the faculty of education in Istanbul, Türkiye.
I have been teaching an educational sciences course at the intersection of Instructional Design and Instructional Technologies and Materials Design (EDS 206) at the Department of Mathematics Education (Grade 5-8), MEF University, Istanbul, Türkiye for 2 years. MEF University is known as the first fully flipped university in the world. You can find out more about the course at the end of this blog post.
This semester, additionally, we had a new visitor to this course. ChatGPT! Yes. Let’s share our experiences in this course.
Using ChatGPT in an educational technology course
I heard that ChatGPT, developed by Artificial Intelligence Developer Open AI, was released as a prototype on November 30th, 2022. I noticed that it attracted people’s attention in a short period of time with its detailed justifications and understandable answers in many fields of information. Many instructional technologists, educational scientists, and even linguists from Türkiye have started using it. It has become popular in our country as well as all over the world.
As a Ph.D. holder of educational sciences and a mathematics teacher; based on my limited experience, I can describe ChatGPT as a companion. Although the database has kept its information until the last updated date, it provides us with companionship in terms of sharing basic, responding fact-based prompts, and comprehensive information. Users must, of course, be aware of the issues that have been raised about the accuracy of the AI too (or see the impact of AI for more information).
Despite this caveat, when I look at it from the perspective of an educator, I believe that teacher candidates can benefit from ChatGPT, when used for the right purposes.
In the EDS 206 course, I demonstrated ChatGPT for a week. Then, I allowed the teacher candidates to experience it for themselves. Some of them asked ChatGPT to talk about common misconceptions made by middle school students in fractions in mathematics, and some of them asked for sample questions of their lesson plan preparation. While discovering ChatGPT, they also learned new instructional design models. They put into practice what they learned in our course while interacting with it. For the accuracy of the information, they had to compare what they learned in the course with the information provided by ChatGPT. At this level, they also started to use their high-level cognitive skills. In their article writing assignments, they were free to use ChatGPT, as long as they referenced appropriately.
To sum up, by following the correct instructions, we teacher educators, can admit ChatGPT as a mentor somewhere in a teacher education program. Nevertheless, it should be used as a means, not an end.
Students’ experiences using ChatGPT
After the ChatGPT experience, I asked my students: “Can you share with me in a paragraph your first experience with ChatGPT in the EDS 206 course, and explain whether it is useful and how your learning experiences in the faculty can get benefit from it?” I made a thematic analysis of their general ideas and initial thoughts. According to the findings of the thematic analysis, I inferenced the following categories.
- Junior-year teacher candidates, studying in the faculty of education and a flipped university, were introduced to ChatGPT for the first time in this course. They were aware that ChatGPT is an up-to-date, innovative, and popular AI-based tool and they gained the specific awareness.
“I think #ChatGPT is a nice artificial intelligence application for people who are researchers and curious. As a teacher candidate, I was introduced to ChatGPT for the first time in EDS206 class and I saw the benefits of the application. During the lesson, my group mates and I experienced that ChatGPT can translate between languages, solve mathematical equations, and offer various suggestions on the subject….”
“I was introduced to the ChatGPT application in the EDS 206 course. In the lesson, we sought an answer to the question of how to use the ChatGPT application in education. We asked the ChatGPT application to develop a training model.”
- All of them found ChatGPT useful for their learning. They see it as a privileged step of being an innovative teacher. When they asked questions regarding maths education, lesson planning, teaching methods etc, ChatGPT provided them with creative and useful examples. For instance:
“…We got surprising results. We discussed these results in class. I think the answers will be useful and effective. I think the most useful feature of the ChatGPT application is that it gives creative and useful examples for desired situations….”
“…While we were experiencing ChatGPT, when we asked “What is the most appropriate teaching model that can be applied on the subject of fractions in mathematics?”, it brought out various models. Although the question we asked was very specific, it brought out more than one model and, most importantly, it explained the focus points of these models with them….”
“…. I wanted to develop a material on “Factors and Multiples” within the scope of the EDS206 course. I wanted to add examples from daily life to my material. I asked ChatGPT to provide me with examples, and source books/sites on this subject. I was redirected to many pages. When we want to make a study by analyzing many sources in education and synthesizing these sources; I can say that ChatGPT is very useful to work step by step.…” (Female, senior year teacher candidate)
- Almost all of the teacher candidates emphasized that ChatGPT encouraged them to use higher-order thinking skills. For example, they stated that they used cognitive skills such as analysis, synthesis, interpretation, and discussion together in the flipped class.
“….When we want to make a study by analyzing many sources in education and synthesizing these sources, I can say that ChatGPT is very useful to work step by step. On the other hand, I can say that it provides ease of learning and analyzing many pieces of literature for students. I can say that individuals who will produce a new study will have the chance to design a roadmap for basic errors, to access the materials to be used here, and to design a synthesized version of many sources if they wish. For this reason, I can say that it also provides a lot of convenience in the production of new works.”
“…. When we further advanced our question and asked it to choose one of these models and create a lesson plan that suited us, its answer really impressed me. Determining the necessary materials, which sections we will divide the lesson into, how many minutes these sections will take, and what we will do in them were explained in detail…”
- On the other hand, only a few of them asserted the possible negative aspects of ChatGPT. Since it depends on machine learning and Artificial Intelligence, the accuracy and validity of the information given by ChatGPT must be tested and controlled from other scientific sources.
“…. Thanks to the information data in ChatGPT, it is a very useful application that allows us to save time by extracting logical answers in the context of cause and effect. If I take a negative aspect, it should not be forgotten that this is an artificial intelligence, if important information research is being conducted, ChatGPT’s responses should definitely be verified with other sources.” (Female, senior year teacher candidate)
Final thoughts
Last but not least, according to my short-term and unique experience regarding ChatGPT, I feel that the contribution of ChatGPT to teacher education is emerging. However, ethical issues should always keep the minds occupied. While discussing the benefits, the critical points and probable negative aspects should be paid attention by the instructors and teacher candidates. We think that ChatGPT will continue to be like a companion that provides motivation during individual learning or unguided instruction, and saves time – as long as it comes from the primary right academic source.
Key Messages
- Teacher candidates can benefit from ChatGPT, when used for the right purposes
- Teaching students reported that they found ChatGPT useful for learning, and saw it as evidence of being an innovative teacher
- ChatGPT encouraged teacher candidates to use higher order thinking skills such as analysis, synthesis, interpretation, and discussion
- Students should be aware of the limitations of tools such as AI and the importance of verifying the information provided with other sources
- The use of AI tools in teacher education is still emerging, and critical points should be considered by instructors and teacher candidates
About the educational science course
The educational sciences course sits at the intersection of Instructional Design and Instructional Technologies and Materials Design (EDS 206) at the Department of Mathematics Education (Grade 5-8), MEF University, Istanbul, Türkiye.
Upon successful completion of this course, students [aka teacher candidates] are expected to be able to:
- explore various ways of thinking about the use of technology in education
- demonstrate how to use a variety of multimedia tools to enrich learning opportunities
- identify appropriate teaching methods and electronic media to support objective-based lessons
- design learning experiences that engage learners in individual and collaborative learning activities
- create electronic multimedia to support specific learning objectives
- use technology to represent topics or concepts in a static or interactive format.
I have been offering the course with an active learning environment both in COVID-19 pandemic times and now in a hybrid format. Teacher candidates apply what they have learned about weekly instructional technological tools, participate in pre-class/individual space and in-class/group space experiences, share their experiences and thoughts during flipped class activities, sometimes evaluate themselves, collaborate, and reflect while learning instructional design theories and practicum with material design.
At the beginning of the semester, the teacher candidates are assigned middle school mathematics content from the national mathematics education curriculum. They learn to design digital materials in order to improve their digital competencies. For example, Bubbl.us, Kahoot, Desmos, Geogebra. They prepare teaching materials for 6th grade students using the digital tools they learn about in the EDS206 related to the mathematics topic they were assigned. However, they design not only independent teaching and learning materials, but also instructional design models and so learn to integrate their digital materials into their ID models.
For more information about EDS 206 please do not hesitate to contact me.
On AI and accuracy
The field of Artificial Intelligence is changing rapidly, and it can be difficult to keep up with the current situation. Here are some articles that we found when this blog post was published.
ChatGPT: Everything you need to know about OpenAI’s GPT-4 tool
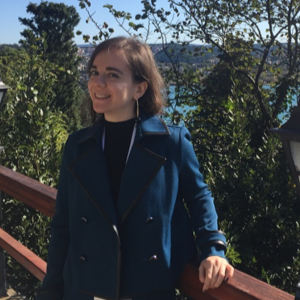
Dr Bengi Birgili
Research Assistant in the Mathematics Education Department at MEF University, Istanbul.
Dr Bengi Birgili is a research assistant in the Mathematics Education Department at MEF University, Istanbul. She experienced in research at the University of Vienna. In 2022, she received her PhD from the Department of Educational Sciences Curriculum and Instruction Program at Middle East Technical University (METU), Ankara. Her research interests focus on curriculum development and evaluation, instructional design, in-class assessment. She received the Emerging Researchers Bursary Winners award at ECER 2017 for her paper titled “A Metacognitive Perspective to Open-Ended Questions vs. Multiple-Choice.”
In 2020, a co-authored research became one of the 4 accepted studies among Early-Career Scholars awarded by the International Testing Commission (ITC) Young Scholar Committee in the UK [Postponed to 2021 Colloquium due to COVID-19].
In Jan 2020, she completed the Elements of AI certification offered by the University of Helsinki.
Researchgate:https://www.researchgate.net/profile/Bengi-Birgili-2
Twitter: @bengibirgili
Linkedin: https://www.linkedin.com/in/bengibirgili/
ORCID:https://orcid.org/0000-0002-2990-6717
Medium: https://bengibirgili.medium.com